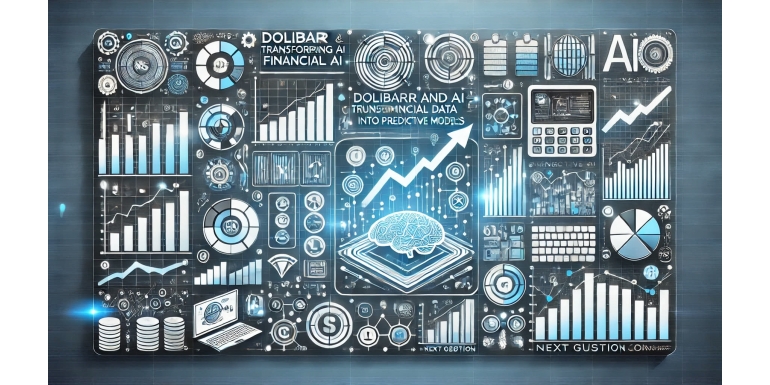
In an increasingly digital world, financial data management has become a top priority for businesses of all sizes. Small and medium-sized enterprises (SMEs) in particular are looking for ways to improve efficiency by automating processes while optimizing decision-making. Dolibarr, a flexible open-source ERP, meets these needs with robust financial management features. However, with the emergence of generative artificial intelligence (Generative AI), a new chapter opens up in how Dolibarr can transform financial data into predictive models.
In this article, we will explore how integrating Generative AI into Dolibarr can revolutionize financial data management and why this innovation is crucial for enhancing the accuracy of predictive financial models, enabling businesses to make data-driven decisions.
What is Generative AI?
Generative AI is a type of artificial intelligence that can generate content from existing data. It uses deep learning models, such as neural networks, to analyze vast amounts of data and generate predictions or new information. Generative AI is applied in various fields, from art creation to text generation, and more recently, financial data analysis.
In an ERP context, generative AI can be used to analyze historical financial transactions, identify trends and anomalies, and generate predictive models to help businesses forecast cash flow, future revenues, and financing needs.
Dolibarr and Generative AI: A Winning Synergy
1. Automation and Financial Forecasting
The integration of Generative AI into Dolibarr allows businesses to automate the analysis of their financial data. Given the vast amounts of data that ERP systems collect, generative AI can leverage this information to predict future revenues, cash flows, and purchasing trends.
- Practical example: An SME using Dolibarr to manage its invoices and payments can integrate a generative AI module to predict low cash flow periods based on customer payment history and anticipated future expenses.
This enables financial managers to plan financing strategies in advance, avoid liquidity issues, and better manage resources.
2. Data-Driven Decision Making Based on Predictive Models
The predictive models generated by AI in Dolibarr provide managers with greater visibility into future performance. For example, they can accurately forecast revenue trends based on seasonal patterns, economic events, or customer behavior.
- Practical example: By analyzing historical sales data, generative AI in Dolibarr can predict which months will be most profitable for a particular business. This allows the company to tailor its marketing campaigns and sales strategies accordingly.
Additionally, these predictive models can be adjusted in real-time, ensuring that financial forecasts are always up to date with new data.
3. Anomaly Detection and Fraud Prevention
Generative AI is particularly effective at identifying anomalies in financial data. By learning from a company's normal financial patterns, AI can detect any unusual, potentially fraudulent, or erroneous transactions.
- Practical example: A company using Dolibarr to manage its expenses can benefit from a generative AI system capable of flagging suspicious transactions, such as unusually high amounts or payments made at odd times. This helps prevent fraud and avoid costly accounting mistakes.
Early anomaly detection can significantly reduce financial risks and enhance the security of accounting data.
4. Optimized Inventory and Cash Flow Management
Beyond revenue forecasting, generative AI in Dolibarr can also help manage inventory effectively by analyzing customer purchasing trends and predicting product needs. This allows businesses to avoid overstocking or stock shortages while optimizing cash flow.
- Practical example: A retail company using Dolibarr to manage its inventory could see the generative AI anticipate peaks in demand for certain products based on sales history. This would allow the company to plan its orders in advance and avoid product shortages.
Concrete Benefits of Generative AI for Dolibarr
1. Cost Reduction and Improved Efficiency
By automating financial data analysis, generative AI allows businesses to save time and reduce human errors. Traditional manual processes, such as data entry and financial reporting, can be automated, reducing administrative costs and improving data accuracy.
Additionally, automation allows financial managers to focus on more strategic tasks, such as growth planning or investment optimization.
2. Anticipating Financial Problems
One of the main advantages of using predictive models is the ability to anticipate problems before they arise. For example, if a company is at risk of facing liquidity problems in the coming months, predictive models can signal this risk well in advance, allowing the company to take corrective measures (such as seeking financing or reducing costs).
3. Tailoring Models to Specific Needs
Generative AI in Dolibarr is not a one-size-fits-all solution. It can be tailored to meet the specific needs of each business. For example, a manufacturing company might use predictive models to optimize inventory management, while a service-based company could use it to analyze billing trends and customer payment habits.
Challenges of Integrating Generative AI into Dolibarr
1. Implementation Complexity
Although integrating generative AI into an ERP like Dolibarr offers numerous benefits, it can also be complex to implement, especially in terms of algorithm configuration and managing large data volumes. Therefore, it’s essential for businesses to have qualified technical resources to fully leverage this technology.
2. Data Security
Using AI often involves working with large amounts of sensitive data, particularly in the financial sector. Therefore, businesses must ensure that their data is protected by using advanced security protocols, ensuring that financial information remains confidential and safeguarded against cyberattacks.
3. User Training
To get the most out of generative AI capabilities in Dolibarr, users must be trained to correctly interpret the results of predictive models. Financial managers and decision-makers need to understand how to use this information to optimize their decision-making processes.
Conclusion
Generative AI represents a major technological advancement for managing financial data within ERPs like Dolibarr. By automating data analysis, creating predictive models, and identifying anomalies, AI enables businesses to make more informed decisions and manage their finances efficiently.
With Dolibarr, SMEs can now benefit from the same predictive tools used by large enterprises, while enjoying the flexibility and accessibility of an open-source solution. However, to maximize the benefits of this technology, overcoming the challenges related to integration and data security is essential.